The Rise of Implementation Science
The practices no longer require research to demonstrate efficacy and effectiveness; as a practical matter, everything that needs to be known is already known. Furthermore, translation of these research findings into actions that can be used in practice is very simple. In addition, because the actions are not prohibitively expensive, cost is not an obstacle, and in fact, cost-effectiveness could be advanced as one more reason for their widespread adoption. Yet their application in the real world is not what it should be, and we need to find out why and to try new approaches to change this situation. (Lenfant, 2003, p. 871)
In this oft-cited editorial, Lenfant makes a compelling case for improving our approach to the application and adoption of research evidence in order to increase return on investments in research (more than US $250 billion invested in the NIH since 1950). Lenfant (2003) provides multiple examples to highlight the prevalent issue of research findings being “lost in translation” somewhere on the “highway” from research to practice. He reports how beta-blockers (shown to be effective for patients recovering from myocardial infarction) and aspirin (shown to be effective for treating unstable angina and secondary prevention of myocardial infarction) were prescribed for only 62% and 33% of eligible patients, respectively. Similar statistics emerge from global health, where only 35% of young children were sleeping under insecticide-treated bed nets in 2010, and nearly 14,000 people living in Sub-Saharan Africa and South Asia died daily from preventable, treatable diseases (Panisset et al., 2012). These statistics converge to reinforce the same message: We know what works, but this knowledge is not successfully implemented in practice.
The imperative to attend to implementation process and effectiveness in addition to intervention effectiveness has emerged over the last two decades in the face of growing recognition that effective practices and treatments do not passively make their way into routine practice. Implementation is not a simple, linear process; rather, it is a highly complex, multi-stage, iterative, multifactorial process that requires distinct expertise and capacity (Brehaut & Eva, 2012). Implementation must be intentional, explicit, and systematic. Emerging research has illustrated that implementation effectiveness is as important as the effectiveness of the evidence that is being implemented, and a strong, positive relationship exists between implementation quality and treatment outcomes (Durlak & DuPre, 2008).
As a branch of KT, implementation science is concerned with facilitating practice, behavior and/or policy change and has emerged as a substantive area of scientific inquiry seeking to remedy the “know-do” or implementation gap. Defined as the “scientific study of methods to promote the systematic uptake of research findings and other evidence-based practices into routine practice, and, hence, to improve the quality and effectiveness of health services” (Eccles & Mittman, 2006, p. 1), implementation science is a global undertaking. The first dedicated peer-reviewed journal for this field—Implementation Science—emerged in 2006, with numerous other peer-reviewed journals dedicating special issues and sections to the implementation of evidence-based practices since 2014 (e.g., Evidence & Policy). Implementation Research and Practice is a new online-only journal focused on implementation in behavioral health from the Society for Implementation Research Collaboration (SIRC), and Implementation Science Communications is an official companion journal to Implementation Science, with a focus on research relevant to the systematic study of approaches to foster uptake of evidence based practices and policies that affect health care delivery and health outcomes, in clinical, organizational, or policy contexts.
Although still somewhat nascent, IS research and practice have developed rapidly. Early implementation research focused heavily on identifying gaps in the use of evidence-based practices and barriers and facilitators to the uptake of innovations into practice. More recently, implementation research has concentrated on developing, revising, extending, and evaluating theories and frameworks, and testing effective strategies and processes for implementation (see below). In addition to these “evolutionary leaps” (Bauer et al., 2015), the IS field has defined and refined its research designs (Curran, Bauer, Mittman, Pyne, & Stetler, 2012), methods and measurement (Lewis et al., 2015), outcomes (Proctor et al., 2011), and reporting standards (Pinnock et al., 2017a, 2017b).
Evolution in Research Design for Implementation Studies
Before the rise of implementation research, KT research was commonly based on randomized controlled trial (RCT) designs to determine the effectiveness of particular KT strategies in changing the behavior of health care practitioners (see Grol & Grimshaw, 2003). During this time, the focus was on KT strategies (what we would now refer to as implementation strategies) and their impact on individual behavior. Fundamental to the RCT research design is the control of seemingly extraneous variables. As research evolved, determinant implementation frameworks identified a range of factors associated with successful implementation that fundamentally shifted our view of the so-called extraneous nature of these variables (e.g., process, inner and outer setting factors). Study designs for implementation have expanded to include a wider range of randomized, quasi-experimental, experimental, and mixed methods approaches (for a good review, see https://impsciuw.org/implementation-science/research/designing-is-research/).
The key processes involved in guiding implementation emerged in several models and frameworks. It also became evident that conducting efficacy, effectiveness and implementation research in a linear manner was inefficient. In light of this, Curran et al. (2012) adapted existing research designs to the field of IS and proposed three types of hybrid effectiveness-implementation trial designs (types 1, 2, and 3). These are described as “hybrid” designs because they simultaneously examine both the effectiveness of the evidence-based treatment and the implementation approach utilized to put the treatment into practice.
The three types of designs differ in the emphasis placed on primarily testing the effectiveness of the evidence-based treatment (type 1), the implementation strategy (type 3), or both (type 2). The advantage of these designs is that they allow for systematically examining both implementation and treatment effectiveness with consideration of the level of evidence for the intervention. As such, hybrid effectiveness-implementation trial designs are more efficient and have the potential to identify important treatment-implementation interactions and enhance treatment delivery in real-world settings.
Another common characteristic of early implementation studies was their nearly exclusive focus on exploring patient- or system-level outcomes. This focus left out consideration of key factors that can facilitate or hinder implementation such as context, implementation process, and implementation outcomes. Specifically, the emerging focus on implementation outcomes is key to understanding clinical outcomes, relative to what works in practice and behavior change (Proctor et al., 2011).
Implementation outcomes are distinct from service outcomes (efficiency, safety, equity, patient-centeredness, timeliness) and client outcomes (satisfaction, function, and symptomatology). Implementation outcomes are defined as the effects of activities undertaken to implement a program and include acceptability, adoption, appropriateness, feasibility, fidelity, implementation cost, penetration, and sustainability. Measuring implementation outcomes in addition to client or service system outcomes is crucial for distinguishing effective or ineffective programs that are well or poorly implemented. Recent work in the United States is exploring measures associated with implementation outcomes (e.g., Lewis et al., 2015).
Finally, complete and accurate reporting of implementation research arguably contributes to the improved translation of research into practice, ensuring consistency in conducting and reporting implementation research, and building on earlier work in a meaningful and transparent way. To this end, the Standards for Reporting Implementation Studies (StaRI) propose requirements for an extensive description of context, implementation strategies, and interventions, as well as reporting on a broad range of effectiveness, process, and health economic outcomes (Pinnock et al., 2017a, 2017b). Recently published, the challenge moving forward will be to disseminate and implement these standards in the academic community, by involving journal editors and requesting use of these standards for submitted publications, as is often the case for other standards such as CONSORT (http://www.consort-statement.org/). Application of the StaRI standards may pose further challenges because it will require its own implementation, that is, behavior change on the part of researchers and adaptations for journals to allow for longer papers and/or permit additional files.
Dissemination and Implementation Categorizations
In 2012, Tabak and colleagues’ narrative review of dissemination and implementation (D&I) models characterized three key characteristics: (1) construct flexibility; (2) degree of dissemination or implementation involved; and (3) the level at which the TMF operates (i.e., socio-ecological framework [SEF] level) (Table 3).
Table 3. Overview of Tabak et al.’s (2012) Categorization of D&I Research
1. Construct flexibility | The degree of flexibility of a model’s constructs. Broad models contain loosely defined constructs that allow greater flexibility to apply the model to a wide array of D&I activities and contexts. Operational Models provide detailed, step-by-step actions for completion of D&I activities. |
---|---|
2. A focus on dissemination or implementation activities themselves |
Models were further categorized on a continuum from dissemination (the active approach of spreading evidence-based interventions to the target audience via determined channels) to implementation (the process of putting into use or integrating evidence-based interventions within a setting). Models informing this category fall along this spectrum from dissemination to implementation. D-only; D > I; D = I; I > D; I-only |
3. SEF level |
Classify models based on the level with which they operate. D&I strategies can focus on change at a specific level (i.e., clinician or organization) or cut across a variety of levels (individual, community, organizational, system, policy). |
Source. Adapted with permission from Tabak et al. (2012).
Tabak’s itemized list of approximately 60 TMFs draws attention to their conceptual flexibility for application across contexts, the focus on dissemination or implementation (or both), and the level at which the TMF operates. Based on this categorization, Table 4 highlights a few select TMFs that KT scholarship has widely applied.
Table 4. Selected TMFs Identified Through Tabak et al.’s (2012) Categorization in D&I Research
TMF | Authors | Overall Aim | D and/or I |
Broad or Operational | Levels of Analysis |
---|---|---|---|---|---|
Diffusion of Innovation Theory | Rogers (2003) | Seeks to explain how, why, and at what rate knowledge and evidence spread. | D-only | Broad | Individual Community Organization |
Streams of Policy Process | Kingdon (1984, 2010) | Provides an overview of the stages in the policy process. | D-only | Fairly broad | System Community Organization Policy |
Research Knowledge Infrastructure | Ellen et al. (2011) Lavis et al. (2006) |
Reflects on the implementation of research knowledge infrastructure (i.e., interventions, tools). | D > I | Operational | Community Organization Individual Policy |
The Reach, Effectiveness, Adoption, Implementation, and Maintenance (RE-AIM) Framework | Glasgow et al. (1999) | Provides a series of stages to guide implementers from research planning to evaluation and reporting. | D = I | Operational | Community Organization Individual |
Ottawa Model of Research Use | Logan & Graham (1998, 2010) | Guides implementation of innovation in six steps focusing on context and innovation, identifying barriers and facilitators, and evaluation. | D = I | Operational | Community Organization Individual |
The Precede-Proceed Model | Ammerman, Lindquist, Lohr, & Hersey (2002) | Allows working backward from the ultimate goal of the research outcome to inform the intervention or strategy design and lays out evaluation methods for pilot and efficacy studies. | D = I | Operational | Community Organization Individual |
A Six-Step Framework for International Physical Activity Dissemination | Bauman et al. (2006) | Focuses on describing the innovation, assessing the target audience, outlining a communication plan, identifying key stakeholders, analyzing barriers and facilitators, and evaluation. | I > D | Broad (but slightly structured) | System Community Organization Individual Policy |
Promoting Action on Research Implementation in Health Services (PARiHS) | Kitson et al. (2008) Rycroft-Malone (2004) |
Examines interactions between evidence, context, and facilitation in the implementation process. | I-only | Broad (but slightly structured) | Community Organization Individual |
Consolidated Framework for Implementation Research (CFIR) | Damschroder et al. (2009) | Provides a consolidation framework from a systematic review that identifies key intervention and context attributes. | I-only | Operational | Community Organization |
Active Implementation Framework | Fixsen, Naoom, Blase, Friedman, & Wallace (2005) National Implementation Research Network (2008) |
Provides several frameworks on process, including notion of implementation teams and implementation drivers. | I-only | Operational | Community Organization Individual |
Source. Adapted with permission from Tabak et al. (2012).
Taxonomy of Implementation TMFs
In 2015, Nilsen categorized TMFs by process, determinant, and evaluative features associated with implementation alone. In this work, TMFs are organized according to their aim to (1) describe and/or guide the translation process (i.e., process models); (2) understand and/or explain factors that influence implementation outcomes (i.e., determinant frameworks, classic theories, implementation theories); and (3) evaluate various aspects of the implementation process (i.e., evaluation frameworks). Figure 4 offers a visualization of Nilsen’s taxonomy. This conceptualization is particularly useful in clarifying that implementation initiatives require guidance from multiple TMFs, to guide the process, identify factors, and evaluate implementation outcomes. Often, researchers fixate on only one TMF when they should be integrating several to address process, factors, and evaluation. Implementation TMFs are complementary, each addressing a core element of implementation: (1) describing and/or guiding the process of implementation, (2) understanding and/or explaining how the process influences outcomes and (3) evaluating implementation outcomes (see Rabin et al., 2020 interactive webtool).
Figure 3. Adapted Diagrammatic Representation of Nilsen’s Taxonomy for Implementation TMFs
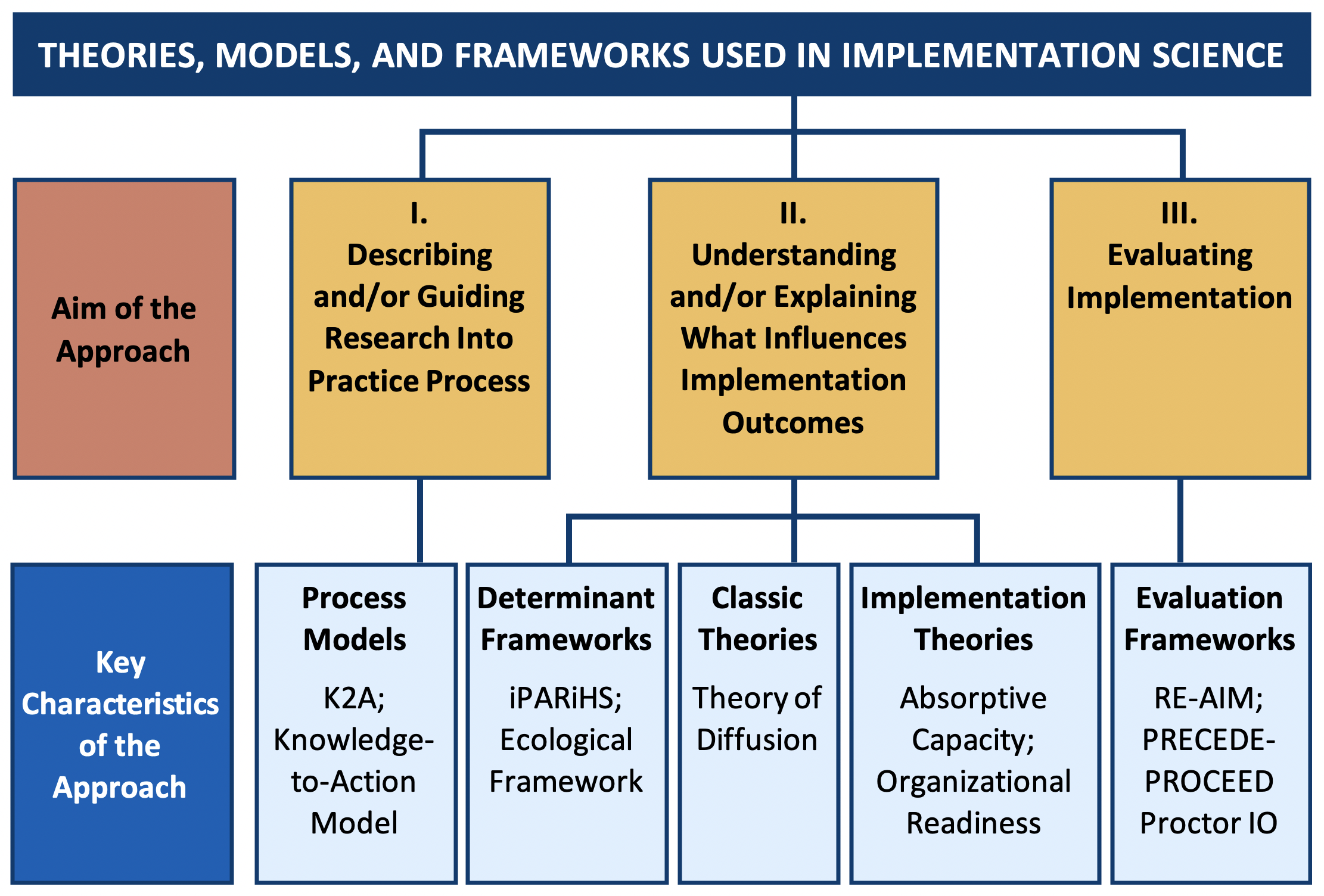
Source. Reprinted with permission (Creative Commons Attribution 4.0 International License) from Nilsen (2015).
Next page: Key Concepts for Knowledge Translation and Implementation